Today's enterprise leaders are well aware that big data is essential to their companies' success, so they collect a lot of it. Unfortunately, most of it isn't ready for prime time. In a recent survey of 200 business intelligence professionals commissioned by the data integration company Xplenty, nearly a third reported spending more than half their time just preparing data for analysis rather than analyzing it and drawing useful conclusions. Some of them said cleaning data took up 90 percent of their work time.
How did our data get to be this unusable? More important, what do we do about it? In this interview, Xplenty founder Yaniv Mor shares some ideas.

The Enterprisers Project (TEP): Why does preparing data take up such a big chunk of data time?
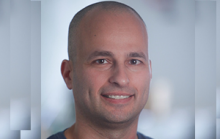
Mor: The proliferation of new data sources and new platforms that generate and collect data — along with the huge increase in data volumes — makes this task even more time consuming than it used to be. For a data person to just grab this data from a variety of sources, located on-premise, in the cloud or on the web, and transform it into a format that's consumable to analytics software (mostly SQL structured) is a big challenge, hence very time-consuming.
TEP: How big of a problem is this?
Mor: More and more companies today understand that they have to become data driven in order to remain competitive. That's not a slogan, that's the reality. So the analysts and the data guys need to sift through the data and provide analytics and insights to the business. Providing analytics is the important part of the job. Data preparation is equally important, but requires a lot of effort and time. What happens is that data professionals spend more of their time on the preparation of data and less on the analytics itself. That's a big business problem.
TEP: Are there particular types of data that are especially challenging to clean and prepare for analysis?
Mor: Data that is not relational usually involves more preparation effort by the analysts than data that is relational. Developers love NoSQL, because it releases them from the effort to maintain a predefined data schema. However, data people need schema in place most of the time because most analytic tools want to consume SQL data. This puts a lot of burden on the data analysts in terms of cleansing and normalizing NoSQL data sets.
TEP: Are there best practices that can create more analytics-ready data?
Mor: Probably the best first step in establishing a best practice here would be a direct line of communication between the developers and the analysts, so when schema changes are required, or when new fields need to be added to the log files that are later being analyzed, at least both parties will know about that and be ready for it.
TEP: As the Internet of Things takes hold across organizations, the amount of data available for processing can grow exponentially. Before addressing the question of how to clean data, is it important to think about which data to collect and which to let go?
Mor: Storage comes very cheap these days and indeed many organizations are adhering to the policy of 'save every bit of data now, we'll deal with it later,' so they will not miss out on any potential data extraction opportunity. It is certainly important to make sure you're only keeping the data you need (or think you'll need) and lose the garbage data, otherwise you will end up swimming, not in a data lake, but rather a data swamp or quicksand.
It should all start with the business defining what it needs to get out of the data. Then based on that, the IT and data teams should map these business requirements with the data sources available and make sure these data sources are kept while other data sources are discarded.
ALSO READ
- HBR article: Retaining your data scientists
- 5 ways CIOs can save time and dig deeper on big data projects
Yaniv Mor is the Founder of Xplenty Ltd. and serves as its Chief Executive Officer. Prior to founding Xplenty, Mor was involved in a multitude of Business Intelligence and data-centric projects with major international companies, and architected state-of-the-art data solutions. He managed the NSW SQL Services practice at Red Rock Consulting, a leading consulting firm in Australia and New Zealand. Mor holds a Bachelor of Science degree in Information Systems Engineering from The Israeli Institute of Technology and a Master’s degree in Business and Technology from the University of NSW, Australia.