As storage gets ever cheaper, many organizations are collecting and keeping unprecedented quantities of data — quantities that will only continue to grow as new systems come online. But how can IT departments deliver data that can actually solve business problems in a timely manner?
In part one of a two-part interview with The Enterprisers Project, Paul Hofmann, chief technology officer of data visualization company Space-Time Insight, offers some ideas.

The Enterprisers Project (TEP): What are the biggest mistakes most IT departments make around data?
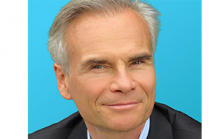
Hofmann: Organizations are collecting and storing data that is generated within their walls (e.g. business and operational data) as well as externally (e.g. currency conversion rates, spot market pricing, weather, traffic, social media activity, etc.). What makes the big data problem so complex is that all this data is siloed. Even when it's combined into one big data system, the applications that access the data are themselves still siloed. While data lakes provide several benefits, having all the data in one physical place does not make business applications and the people that use them any less siloed than before.
Silos are one of the primary reasons organizations are not deriving value from data. The effort for business users to obtain one set of data in a usable format and then manually correlate it with other data in other formats is extreme. It's no wonder that Forrester Research reported that 88 percent of data goes unused.
This problem will be even further exacerbated as organizations deploy and otherwise capture data from a rapidly increasing population of IoT devices.
Going forward, it is critical for IT to move beyond traditional business intelligence approaches that focus on offline reporting and analysis of events that have already occurred. As more data is generated by the Internet of Things, businesses will live and die by their ability to not just respond more quickly to what is happening, but to be proactive in leveraging opportunities and heading off disasters before they occur.
With necessity being the mother of invention, the necessity of breaking down data silos and facilitating real-time or near-real-time decision-making across an organization has given rise to a new category of software called situational intelligence. Situational intelligence combines business intelligence techniques with operational and location intelligence to give businesses a 360-degree operational view. Key to the success of situational intelligence implementations is the application of advanced analytics to large volumes of disparate data and the rich visualization of that data in a single dashboard.
To this end, IT needs to be an enabler for timely and simple access to data. In many cases today it takes weeks or months to provide business users with the data they need to answer pressing questions. Given the pace of today's business environment, that speed of response is not viable and it puts organizations at risk of not being responsive enough to customer needs or competitive enough to stay ahead in the market.
It is also up to corporate leadership to identify the strategic initiatives that require IT's engagement and accountability. These initiatives might focus, for example, on cost reduction, productivity improvements or quality of customer service. In each case, some combination of disparate data, advanced analytics and data visualization will inevitably be required to provide the actionable insights business users require.
TEP: Is it possible to have too much data? What are the problems it poses, and how do you solve them?
Hofmann: The rise of the Chief Data Officer (CDO) role is testament to the need for data governance policies, and active management of those policies includes data retention. Understanding internal needs, external service level agreements, legal and regulatory requirements, accessibility vis-à-vis hot storage and cold storage, to name a few, are without question extremely important in the determination of what data is needed and how long it is needed for.
A number of industry analysts have made the point that organizations that derive value from their data are most likely to succeed. It follows that in order to derive value, the data has to be stored. To determine whether the data is useful and should be stored for a period of time, it is best to start with the known and potential use cases for the data.
Corporate reporting needs frequently require access to historical data, but that data is typically aggregated so it may be possible to store just the aggregations if the volume of source data is too significant. However, that detailed data may be critical to the success of analytics initiatives that rely on an understanding of historical performance to project future outcomes.
TEP: Does this approach apply to IoT-generated data as well?
Hofmann: IoT and real-time applications pose a slightly different set of challenges. It's not just that the volume of data is significant, but also that the demand for timely access to the data requires a different approach to collecting, cleaning and analysis. Where and when the data is stored long-term plays a secondary role to how quickly useful insights can be presented to users. For example, the value of data being generated at 60 times per second from sensors on the electric grid is that operators and automated systems can gain precious seconds to react to an impending failure.
Many organizations face a data quality problem and this adds another dimension to the question of whether there's too much data and what data to keep. The problem is partly one of missing or inaccurate data, but often one of redundant data which is why solid governance policies are so important. The best place to start addressing this issue is to first understand the scope of the problem.
Some companies use analytics and data visualization to help see their way through these quality issues. The simple act of laying assets and other resources out on a map can be extremely beneficial in understanding what data is incorrect or missing, and provides a forum for users across the company to contribute to improving the quality of the data. In addition, analysis of data across systems helps identify redundancies, inconsistencies and gaps in availability of key performance inputs.
There is clearly a thirst for information in almost every organization. It's frustrating to many employees that there is also an endless and inaccessible sea of data to work with. This suggests that IT should shift its emphasis from data storage to providing the ability to harness the data and make it actionable. Once organizations become proficient at deriving value from their data they will be better able to determine the capture, capacity, and retention policies that will benefit the business most.