For many IT operations, big data should really be called "Big Overwhelming Data." Extracting useful information from all the data flowing in from all directions is like trying to get a drink from a fire hydrant. Many companies are coping by creating big data policies that determine which data is captured and analyzed, and which is not useful enough to retain.
That's an understandable approach, but it's the wrong one, according to Paul Hofmann, CTO of data visualization company Space-Time Insight. In the first part of a two-part series, Hofmann explained how data silos often confound IT's attempts to make business use of big data. In this second part, he explains how companies can indeed make business use of all the data that comes their way.
TEP: What's wrong with big data policies that restrict the types and sources of data that companies collect?
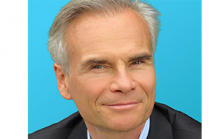
Hofmann: Many organizations actively choose not to collect some data today. In some cases this is because there's no place to store it and sometimes nobody can identify a clear value for the data. One can understand this rationale especially in light of IoT systems that generate exponentially larger volumes of data than those that preceded them. In addition, if all that data is actually collected and stored, how are users going to be able to consume it in a timely fashion or at all?
This view is ultimately short-sighted since the real value to a business is not the collection or storage of data. It is the analysis and understanding of that data that is important. This short-sightedness is born out of the way traditional business intelligence systems work. Data is loaded into a warehouse, reconfigured into aggregations, and the aggregated data is then presented to users. In an IoT world, this approach simply doesn't work or scale.
TEP: Why is that?
Hofmann: First, timeliness is critical. By the time data is loaded into a warehouse and made available to users, opportunities and issues are often long gone or have escalated. Second and more importantly, given the volume and nature of IoT data, it is not appropriate or practical to load it all onto a user's desktop.
Situational intelligence approaches the problem as follows: Data is analyzed as it is streaming in, significantly shortening the time from sensor to decision. In addition, only the data that users need to be concerned with is presented to them. If a rapid response to certain conditions is required, there's no need to burden users with data where those conditions are not met.
IT should realize that data is extremely important to the success of analytics initiatives. Every piece of data history that is abandoned on the roadside could have implications down the road for an organization's ability to understand how and why assets and resources behave in certain ways, and how they might behave in the future.
The ability to capture and process IoT data is table stakes to participate in and benefit from this new data-driven world. With predictions of billions of connected devices coming online in the next few years, opportunities for new revenue streams, new business models and entirely new industries will be revealed. There are numerous "brown field" capabilities that organizations will need to acquire and become proficient in and they will be constrained by the limitations of legacy systems that cannot connect to a variety of sensors and data formats. A lot of real-time processing is needed to unpack data, normalize it, monitor it for specific anomalies and events, commit it to a repository and so on.
TEP: You've said that OT (operational technology, such as that which runs factories or heavy industry) creates major data silo issues. Are there ways for IT leaders to capture this data?
Hofmann: Systems and data that are obscured and not on an organization's grid prove to be a difficult issue to manage. There is of course top-level governance and auditing. However, most organizations need a bridging technique that's not as draconian as governance and auditing. Approaches such as situational intelligence that can handle data from any disparate sources are now available and arm IT with the ability to bridge the IT/OT gap.
For example, users might want to understand which assets (such as machines or pieces of equipment) are currently operating under stress, access the details for those assets, determine whether temperature or other environmental conditions should be considered, assess the impact on service delivery if one of those assets fails, pull up the cost and revenue implications of such a failure, and take action to prevent that scenario from taking place.
To pull this off in the past would have required multiple people accessing multiple systems and manually correlating the data. With situational intelligence, it all takes place in one application, and users in different roles all have access to consistent information as they collaborate to address issues.
TEP: How is this accomplished?
Hofmann: A driver behind situational intelligence is that it aims to overlay existing systems as opposed to replacing systems or duplicating data (which would create even more unwanted silos). An in-memory approach allows IT to build a data storage infrastructure to support a wide array of uses without needing to create analytics-specific warehouses and the like. Tied to this is a concept mentioned above — humans cannot consume too much data and it is critical to use analytics to do the work of identifying the data that requires attention.
We are commonly asked "Is visualization software able to display millions of data points on a map?" While the answer is yes, the question itself is not the one companies should be asking. It is far more important to understand what business problems users are trying to solve and determine what data is required to support those problem-solving efforts.
If a user is trying to understand which of millions of assets are at risk of failure for example, it doesn't make sense to show all the assets on the screen and let the user sort through them. Instead, analytics can identify and prioritize the most critical assets, helping the user focus on the task at hand. With an approach like this, it is possible to derive immediate value from data, justifying further investments in big data and analytics infrastructure.
Quickly prototype potential solutions
TEP: Any other advice you'd give CIOs about dealing with data in today's IT landscape?
Hofmann: IT needs to start thinking about data differently than in the past. The processes and technologies that worked 20 years ago are no longer viable today and without making adjustments to real-time processing and the IoT, IT will inevitably fail to adequately support its internal customers. It's not just about how, when and where data is captured and stored. It's about how, when and where value is derived from that data.
Many of the process changes we've talked about also imply cultural and organizational changes. User expectations and needs are continuing to add to the IT backlog, though often in different ways. The emerging roles of CDO and Chief Analytics Officer suggest that executive sponsorship and support for these new initiatives are essential. This sponsorship also needs to come with an understanding that certain analytics initiatives may not return any results of interest if in fact there's nothing to find.
It's also important to be able to quickly prototype potential solutions. Given how fast technology is evolving, it's common for business users to not know what they want or even what is possible. One of the benefits of an agile approach to implementation is that a straw man can be quickly and collaboratively created, making it easier for all team members to refine requirements into solutions that are useful, usable and valuable.
This is where visual analytics platforms and rapid development solutions can add tremendous value. Benefit can be delivered to the organization with little to no IT burden, freeing IT resources and the CIO to focus on high-priority projects and strategic initiatives.
As Chief Technology Officer at Space-Time Insight, Paul Hofmann, PhD, draws on over twenty years of experience in enterprise software, analytics and machine learning. He has held executive roles at BASF and SAP, where he was VP R&D, and conducted academic research at MIT, Technical University in Munich and Northwestern University. Most recently, Paul served as CTO for Saffron Technology.